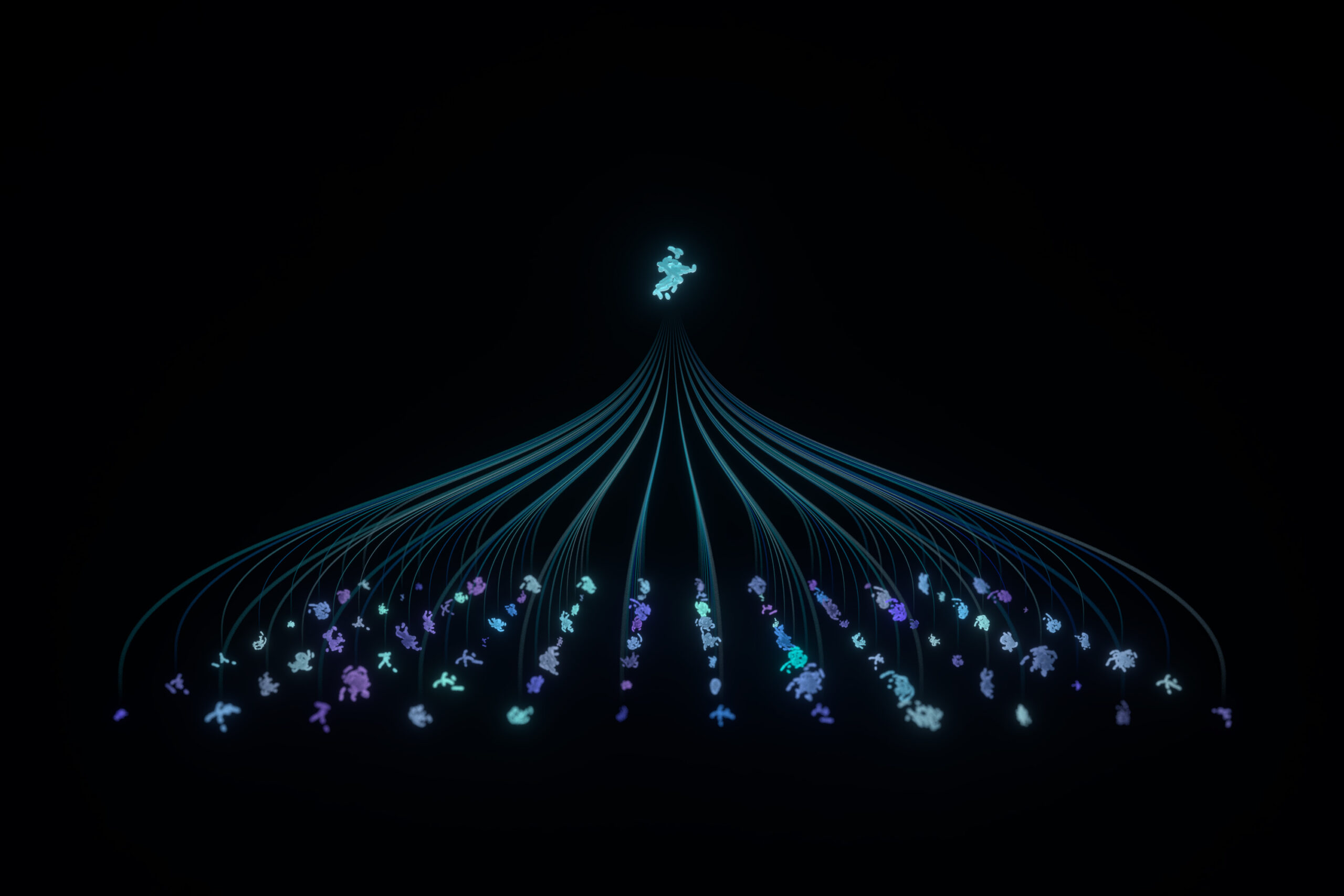
Locus deploys three different flavors of AI and our drug development efforts. The first is machine learning, which I’ll get to in more depth later, but briefly it allows us to use our high throughput automation capability to measure the outcomes of each of millions of different bacteria and phage reactions. These outcomes are then used to train a machine learning model. Which allows for prediction of the performance of quadrillions of phage cocktails. That’s 10 to the 12 or 1000 times more than trillions. This machine learning approach to lead optimization results in a more optimal drug that’s capable of meeting all of the clinical endpoints, and I think it’s one of the key reasons that we’ve succeeded where so many others have failed.
A second way we use AI is to identify optimal insertion sites for recombinant payload expression within the phage genome. For this we use biological natural language processing combined with this concept of pangenomes, which is just a fancy way of saying the entire genomic content of a species. We do this by first creating the pangeome which serves as a reference set of all conserved and variable genomic regions. We then train an NLP model on the genomic sequences and associated expression data, essentially teaching the model the language of DNA, where nucleotides are letters, genes are words, and operons are sentences. The trained model allows us to make predictions on the likelihood of achieving strong payload expression and payload genetic stability for different sites within the phage genome. It’s impracticable to test every one of the hundreds of thousands of sites in the phage genome by engineering in a payload or a reporter gene. But this NLP based approach allows us to do exactly that in silico. This is another key to our success because higher payload expression levels translate into a more effective drug in the clinic.
A third way that we use AI is for the ability to conversationally query the massive database we’ve built over the last eight years. So we’ve set up our own local large language model that converts English language queries into SQL database queries and then returns the best answer for a given prompt. Our scientists can ask questions like. What phage is best able to suppress bacterial strain X? And then get an answer returned in a few seconds. Now that’s a simple example, but the queries can be much more complex and can even involve the use of logic. This is incredibly helpful because it democratizes the company’s historical data, making it instantly available to solve current challenges.
These are a few of the ways we use AI today to develop more effective drugs. But we continue to brainstorm new implementations and to identify new use cases nearly every week.
Please visit the Platform page to learn more about how LOCUS is used to build our engineered, precision phage therapies.